Is usage-based pricing overrated? These 3 YC founders agree (and so do we)
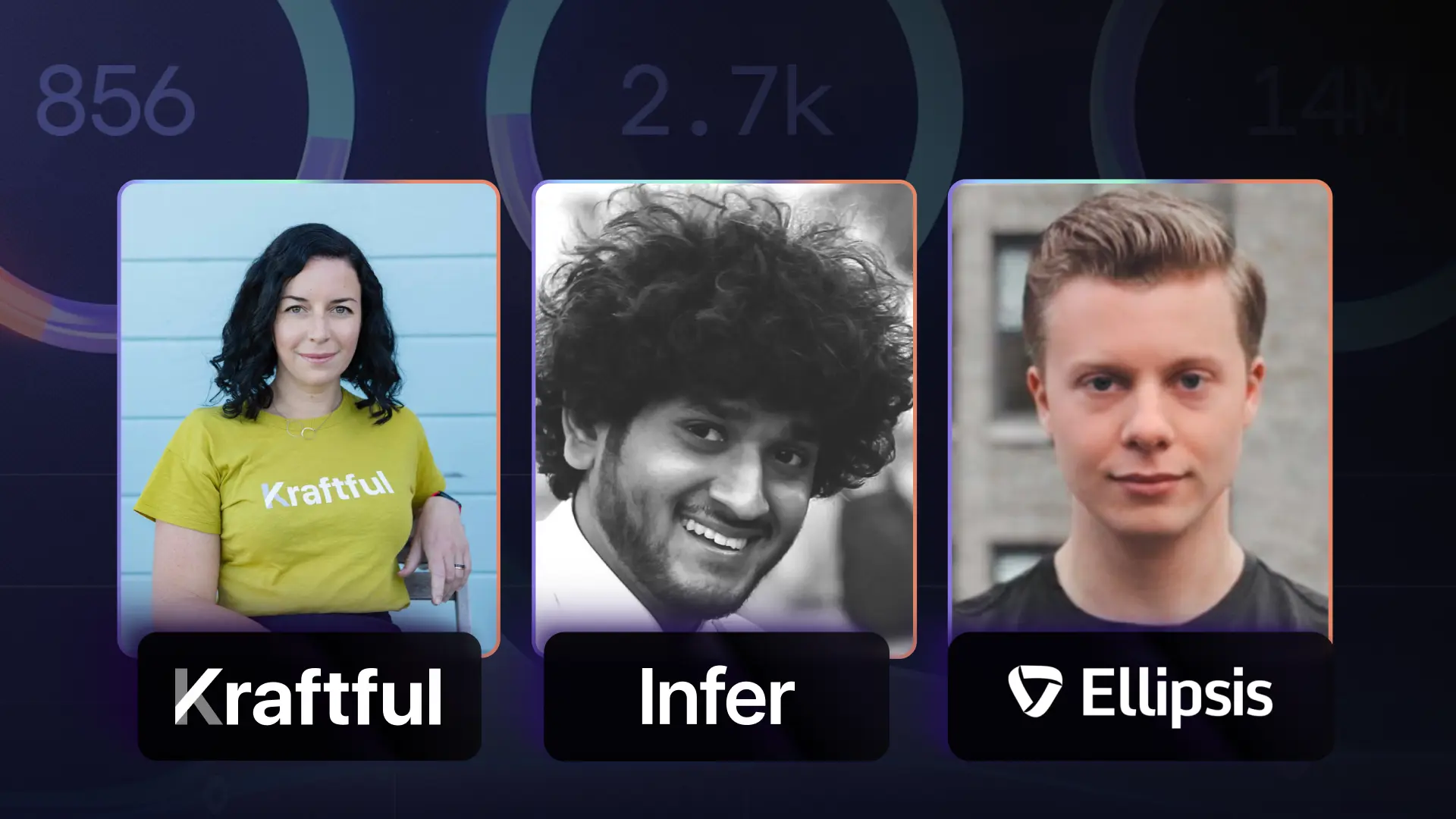
If you love pricing, I’m sorry for you. It means you’re a nerd like me, someone who’s fascinated with the ripple effects of tiny, but fundamental decisions. It means you have to live with the knowledge that nobody in real life cares about your little niche interests.
Luckily, this is the CommandBlogue, where we can both geek out together and know we’re not alone with our weird obsessions.
James recently published an article about how we priced our AI SaaS product (Copilot). But one company’s answers don’t have to be true for everyone, especially if it’s been less than a year.
That’s why I wanted to know more. That’s why I looked for some YC founders building AI products. Over the past weeks, I spoke with the founders of Kraftful, Ellipsis and Infer about AI pricing—how they think about it, which model they ended up with and which other models they considered.
Want to know how YC startups think about AI pricing? Keep reading! If you’re familiar with the unique challenges of pricing AI products, feel free to jump to the examples on the left sidebar. If you want a quick primer, I’ve excerpted James’s AI SaaS pricing article below:
Why AI pricing is so hard
“Traditional SaaS pricing grants (more or less) access for a fixed fee with similarly stable costs. But paid-by-the-token APIs, we could get a difficult scenario: Power users taking the “unlimited” too literally and bankrupting you.
In nerdspeak: AI apps have COGS.
This has spawned much social media chatter in the SaaS community. Should you pay based on usage? Should users foot their own AI bill?”
When each user interaction costs you money, pricing gets trickier. This has led to a lot of discourse on the evolution of pricing in the future. And since startups are the future, who better to tell us all about it than Y Combinator founders? Let’s dive in!
Kraftful — the user feedback copilot
If you’re a product person, you probably love the strategic work, but hate spending hours reading survey results until they look like hieroglyphs. Kraftful (YC S19) aims to fix this.
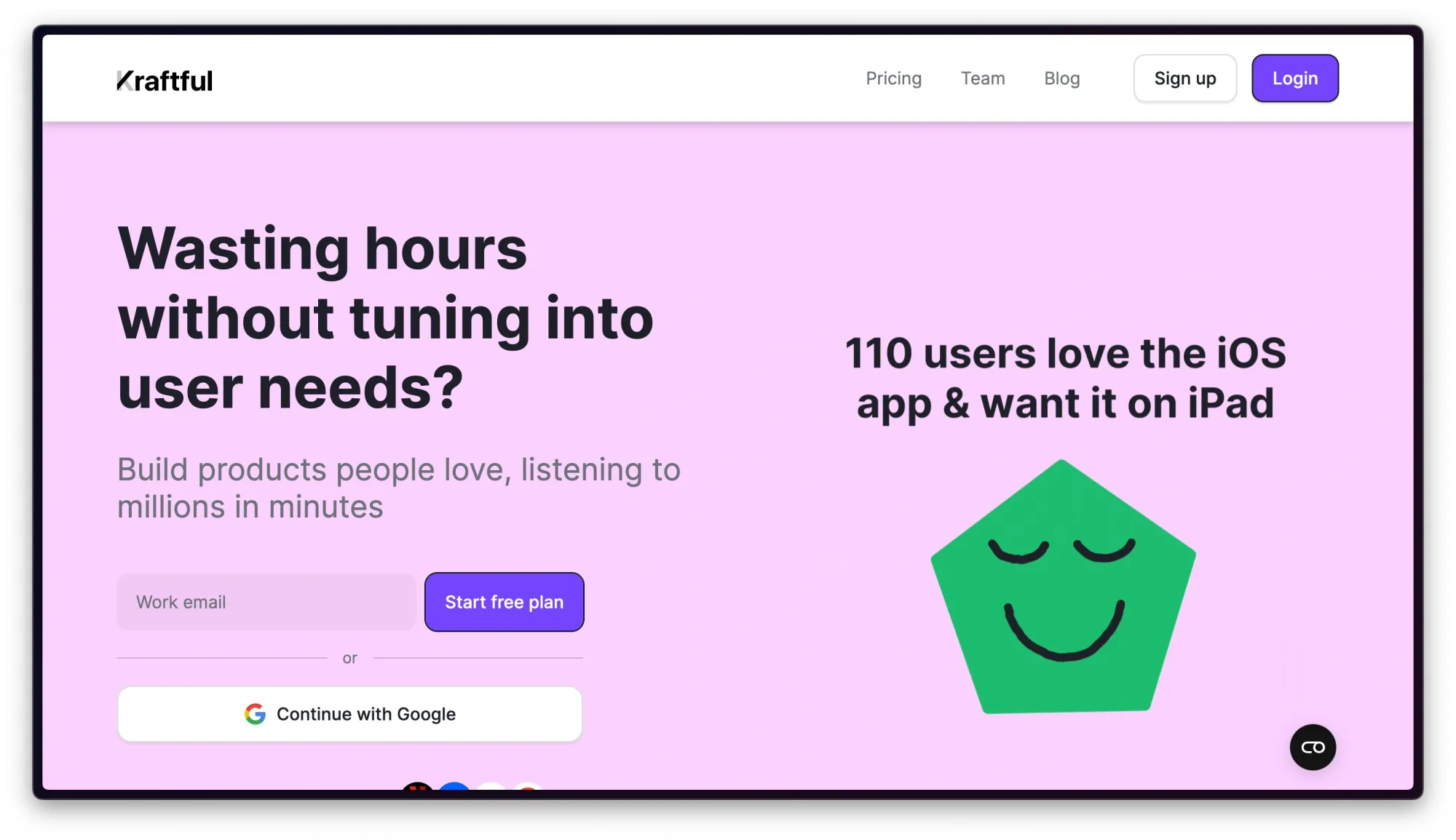
Kraftful is an AI product that takes all the text inputs you deal with and analyzes them with AI. You’ve probably spent countless hours sifting through support tickets, user feedback and other text. Kraftful saves you those hours if you connect one of 30+ feedback sources.
This puts Kraftful into a potentially difficult spot: Some companies have orders of magnitude more feedback to analyze than others (Think of B2C vs. B2B—Consumers share so much more input in public). This means the cost for different customers also varies.
The pricing itself is what James defined as a usage-based subscription: Kraftful customers pay a monthly or yearly fee. This gives them unlimited access to some features and lets them AI-analyze a limited word count. In effect, you buy a certain amount of credits each month, similar to a phone plan that costs $15 each month and includes a certain amount of data.
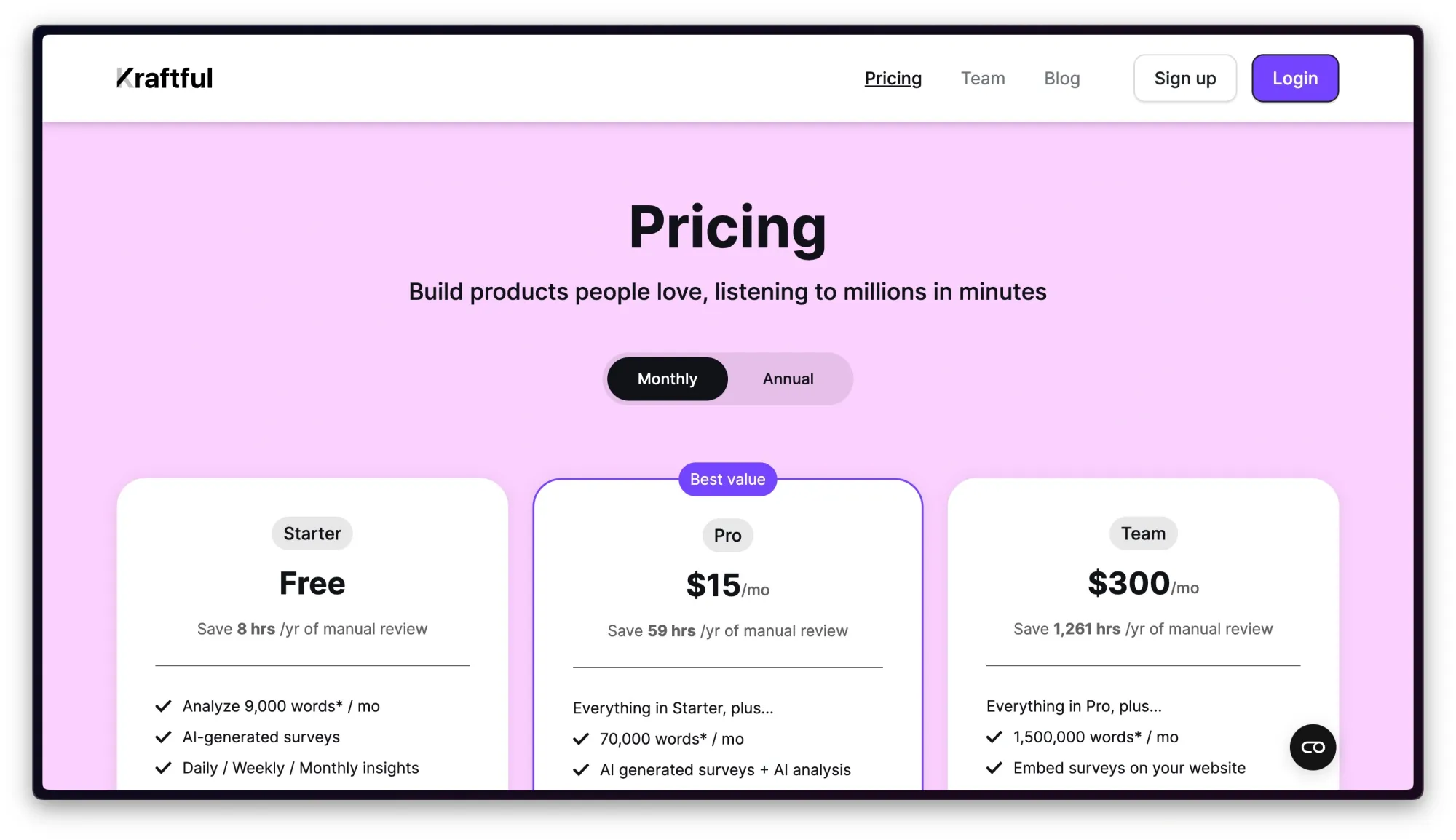
Those hours are what CEO and founder Yana Welinder anchored to when I asked her about Kraftful’s pricing strategy:
“We base our pricing on word count to transparently reflect the time savings our solution provides. For instance, our Team plan saves 1,261 hours of manual review, demonstrating to customers that the cost of using Kraftful is more than 25X lower than manual labor. This clear value translation helps customers understand the financial benefits instantly.”
Kraftful’s quantification in hours reminds me of Sarah Tavel’s Sell Work, Not Software: Imagine you were hiring Kraftful as a person—how would you compensate them for mining through insights in thousands of documents? You’d probably pay them a monthly retainer—not a commission per bullet point created.
That’s why I especially love two insights from Yana here:
- Deriving pricing from value: The biggest benefit Kraftful users get is saving time. This is where Yana’s thinking starts. One of the jobs of pricing is to align incentives—which is easiest when prices are based on value creation.
- Anchoring to human labor: Quantifying value in B2B SaaS is hard, especially for startups. Unless you’re an ads/sales platform that can prove direct ROI, your value will always be more qualitative. The Kraftful team found a way to reframe this: They focus on productivity gains (manual review time saved). That makes it easier to justify any given price. ****
But if you’ve been paying attention to the recent discourse in the SaaS world, you’ve probably heard the predictions of an uptick in usage-based pricing. Yana said they explored the option:
“We've considered usage-based pricing but found its unpredictability deterred potential customers, with some exceeding their budgets unintentionally. This negative experience led us to seek a more predictable and customer-friendly model.”
This is interesting because usage-based pricing is great in theory: People have subscription fatigue, businesses are consolidating costs. Usage-based would seem like a perfect model—you have access to the tool when you need it, but don’t pay when you’re not using it.
Instead, Yana’s response here highlights another important aspect of pricing: The buying experience matters.
And something that costs “???” is hard to buy. You can no longer just enter your credit card data and use the tool, but have to model your expected use to estimate costs. If you work in a bigger company, getting approval a usage-based tool is way harder because the cost isn’t clear.
This is why usage-based pricing makes it so much harder to buy software—even if customers pay more. She described her way of pricing as “more customer-friendly”, which seems ironic since customers might pay more with a subscription.
But a usage-based subscription also includes the benefit that you can offer more output at a lower price: Customers who don’t use up their allowance subsidize those who do, which lets companies offer higher limits at the same price.
Yana views her pricing thoughts as a success:
“Our pricing model has proven effective, as it offers transparent ROI calculations, encouraging customers to upgrade when their data analysis needs grow. This clarity and predictability have made it an easy choice for customers, enhancing their experience and satisfaction with Kraftful.”
I particularly liked Kraftful’s first principles and customer-focused approach. But Kraftful isn’t the only AI YC company (shocker, I know).
Ellipsis: Easier code review (and more) with AI
Ellipsis is a GitHub & GitLab app that automates code review by leaving suggestions, comments and summaries in over 20 languages. If you tag Ellipsis in a pull request, it can also generate code.
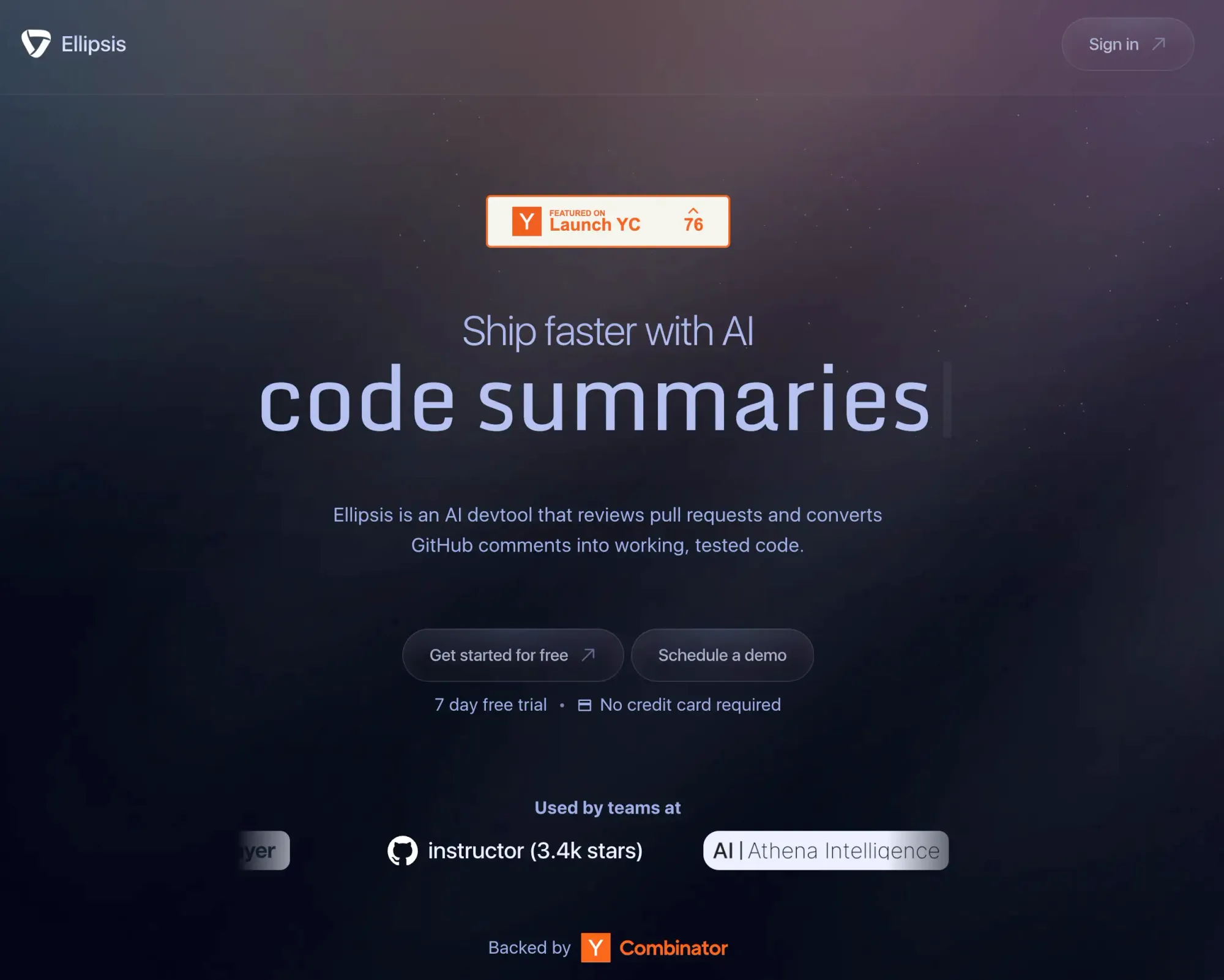
I spoke with co-founder and CTO Nick Bradford about how he and his team think about pricing. He told me that “We started with usage-based pricing, but quickly switched to a per-seat model because that was much more intuitive for customers.”
Usage-based pricing didn’t remain a thought—the team actually pitched it to potential customers:
When you're first launching a product, priority one is to figure out if you’re solving a real problem that people will pay for, so you want to reduce friction and complexity as much as possible, both for the customer and your internal stack. Optimizing pricing comes later.
This is laudable. As an early-stage startup, you want to know what (and if) people are willing to pay for your product. Pricing is usually a high-stakes decision because it’s the foundation of your company’s economic model. But if you don’t have customers yet, pricing becomes a lower-stakes decision. Just testing how people prefer to pay is the better option if the downside is tiny.
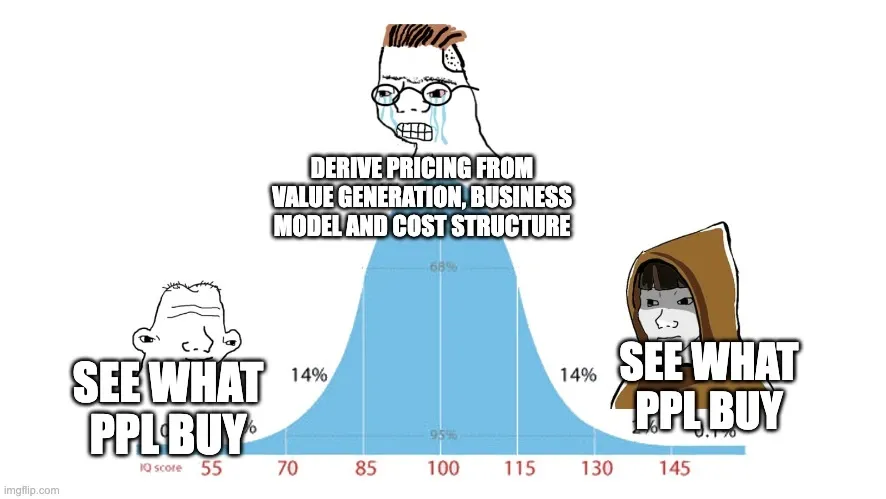
This is similar to what I mentioned with Kraftful: Usage-based pricing makes your product harder to buy, harder to argue for in a bigger company.
So far in this article, we’ve talked about pricing in terms of user/customer experience. But that’s not all you optimize for in pricing. Nick continued:
“We found usage-based to be more popular with hobbyists, students, and open source, because their usage was often unpredictable. In a larger company, the decision-maker has a set budget and needs to decide how much they can allocate to your product. This tends to be fine because larger organizations also have more predictable usage.”
This is what I mentioned above. Buying any product is just easier if it has a price tag because it saves so many headaches internally for your users. If you sell software that helps your customers save time, better save them time buying too. Most leadership-level people prefer paying an extra $500 than 10 extra 1-hour meetings.
Another idea Ellipsis explored is the “bring your own key” model: Have customers create their own API key, then let them enter it. They foot their own AI bill and pay you for the software.
This wasn’t optimal either:
“Bring-your-own-key has a number of problems. It prevents you from iterating quickly on the backend to swap out different model providers. It’s a poor user experience, because it adds friction and forces the customer to think about implementation details. It forces the customer into one specific kind of usage-based pricing you may not want, giving you much less flexibility.
“Bring your own key” seems like it not only makes buying more complicated, but also the user experience itself. It simply creates too many dependencies—what if OpenAI revokes your customer’s API key? That ruins the experience with your product, even though you haven’t made any mistake.
Now, the normal SaaS pricing model comes with a downside: Theoretically, a single customer could bankrupt you by spamming your AI features to the point where you pay millions to OpenAI. I asked Nick whether he worried about customers overusing their app: We’ve had a couple of those already and we just negotiate one-offs with them. There’s not so many that we’ve needed an automated system, but we eventually will.
He says they can afford to be less worried than others because: “Code generation is power law, code reviews is Gaussian”. This is a great example of deriving insights from first principles. A single user might generate a crazy amount of code in one day, but code review is somewhat bounded because nobody is committing 2000 times a day. In Nick’s words: “With code generation, a power user can trivially spin up hundreds or thousands of workflows, which is great, but also follows a very different distribution compared to developers submitting pull requests. We’ve had single users on code generation consume more than the next thousand users combined.”
Nick also mentioned doing custom deals for bigger customers or ones with specific needs, like open source projects. This makes sense. An open source project might need dozens of seats, but most of those will barely be used because most open source development comes from a tiny minority.
This shows that it’s important to be flexible with your pricing model if a customer has (justified) specific needs.
How Infer prices their AI agent product
For my third and final interview, I spoke with Vaibhav Saxena, who co-founded Infer (YC S21). With Infer, end users can build and deploy AI voice agents that respond by considering what they say and how they say(reads their emotions) and also takes actions (think sending email, updating crm etc.)
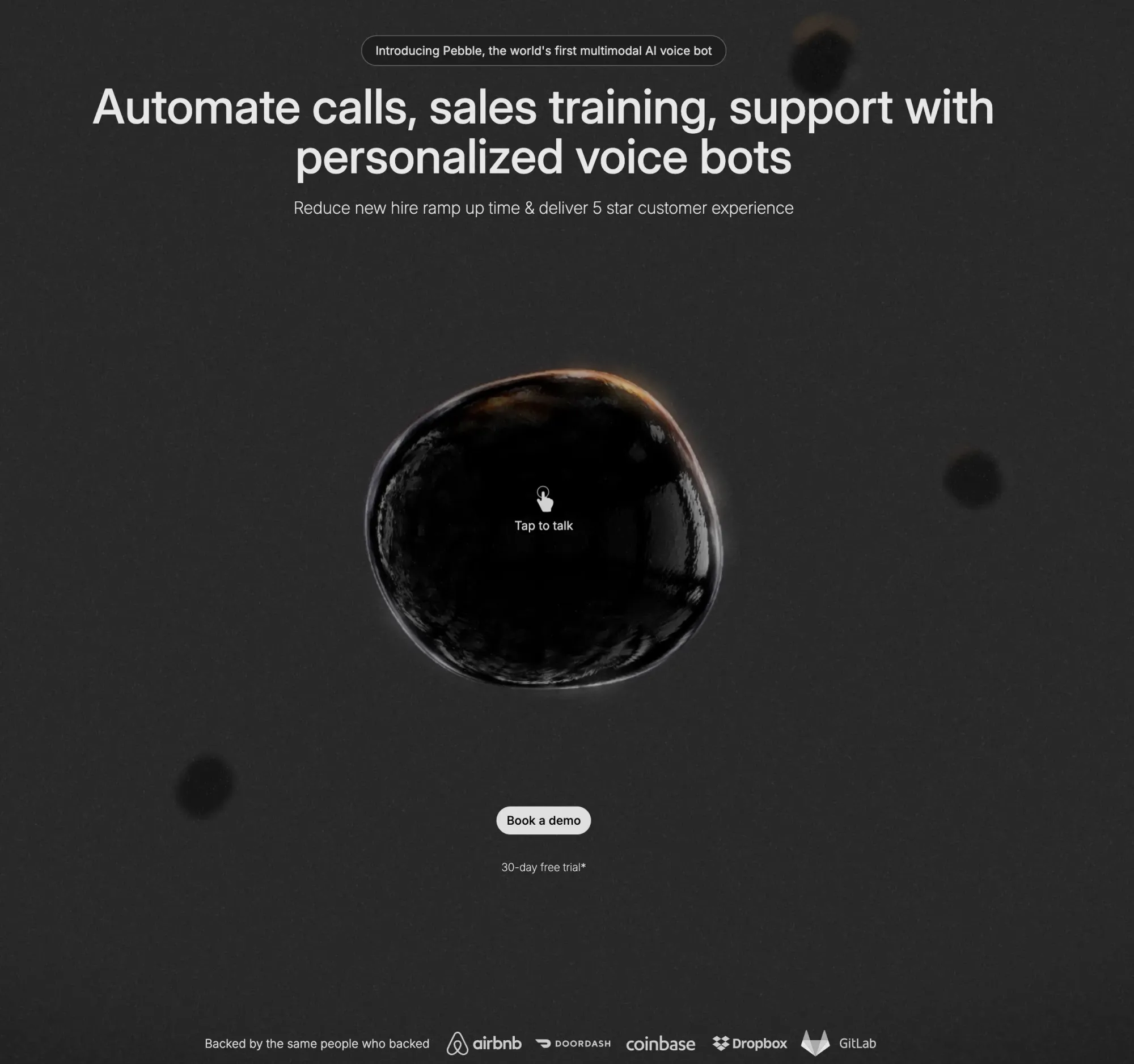
According to the website, Infer is ideal for sales training, lead generation & qualification, customer service and similar customer-facing tasks.
Vaibhav quickly helped me understand that Infer is a very different product from Kraftful and Ellipsis. Both of those tools have low downside if they don’t work, which makes users more likely to be willing to try them out. Infer is different: A botched AI bot voice interaction could mean losing customers. Plus, the AI needs to be trained on proprietary content and deeply integrate with the product.
These are first principles that require a more hands-on approach. The Infer team has perhaps the most complex pricing model:
“We target people who don’t know prompting and APIs and they want us to manage those things for them. Our pricing then becomes a set of fees because we fine-tune an ai model for them. Then we charge a monthly fee and a usage-based pricing.”
The usage-based component works with a minimum consumption model and requires customers to consume at least a certain amount of minutes. This is similar to the credit system Nick mentioned above.
I found the setup fee pricing interesting because it means Infer basically starts with a consulting package. There’s something “Do things that don’t scale” about this: Many founders would insist on building a self-serve motion so they don’t have to do the manual implementation work up front. But eschewing this also means missing out on the learnings that come from talking to customers. Plus, if you can get paid to do it… do it!
When I asked Vaibhav about usage-based pricing (in the “use nothing, pay nothing” way), he shared why he didn’t believe it was the right move for Infer: “We had thought about plain usage-based pricing because other people were doing so. But the biggest problem is what happens when someone uses almost no volume in a given month.”
“That doesn’t help us by any means. We want to be certain of a minimum revenue every month. We do not work with customers who have 10 calls a day. People invest a lot of time to set it up, and that’s not worth it. We can just get a customer who has 30-40 calls a day.”
I liked this answer a lot because it highlighted the value of segmenting and keeping your audience in mind. If you get bad feedback on your pricing from someone you wouldn’t want as a customer, what gives?
Next, I asked him what impact he has seen compared to other pricing models: “Customers are poking us more, asking more questions. There’s a slight initial pain because they give us an initial set of fees.”
This introduces some amount of friction. In this case, that’s a good thing because the setup is so time-intensive and a product like Infer requires deep integration. If Infer was fully product-led, it would most likely end up with a ton of accounts with little activity. The payment incentivizes new customers to stay engaged.
When I asked about his biggest surprise, he told me: “People don’t question subscription fees. They’re questioning usage-based pricing: I ended up negotiating price decreases for minimums. Plus, I can increase subscription prices on a very different thing—we’re providing you dashboards and other things. No objection has come from subscription pricing because everybody’s paying for some kind of subscription.”
But some pricing questions can’t be answered universally. Next, Vaibhav shared some AI voice agent-specific insights: “It’s very similar to the editor-based approach in SaaS. It will be paid per voice agent. We might see customers create an inbound agent, an outbound agent and have different ones for each product they sell.”
Will we just have subscriptions forever?
So far, the most interesting part was that all these YC founders focused on fundamentals and mostly eschewed novel pricing schemes like success-based, usage-based or bring-your-own-key pricing.
Maybe that’s because software pricing has found its logical end point: Recurring revenue is the holy grail and we’re done innovating on pricing. Or maybe it’s because AI affects everything, but not pricing. Maybe AI models get so cheap over time that it’ll look foolish that this was ever a big deal.
I think there’s something important here: None of the YC founders we spoke with are building API only products. Perhaps the most successful AI product is purely usage-based: OpenAI’s API.
And the closer something is to being “raw material”, the more likely you are to make usage-based pricing work. This has been true way before the rise of AI. This is Twilio (which helps enterprises connect with their customers:
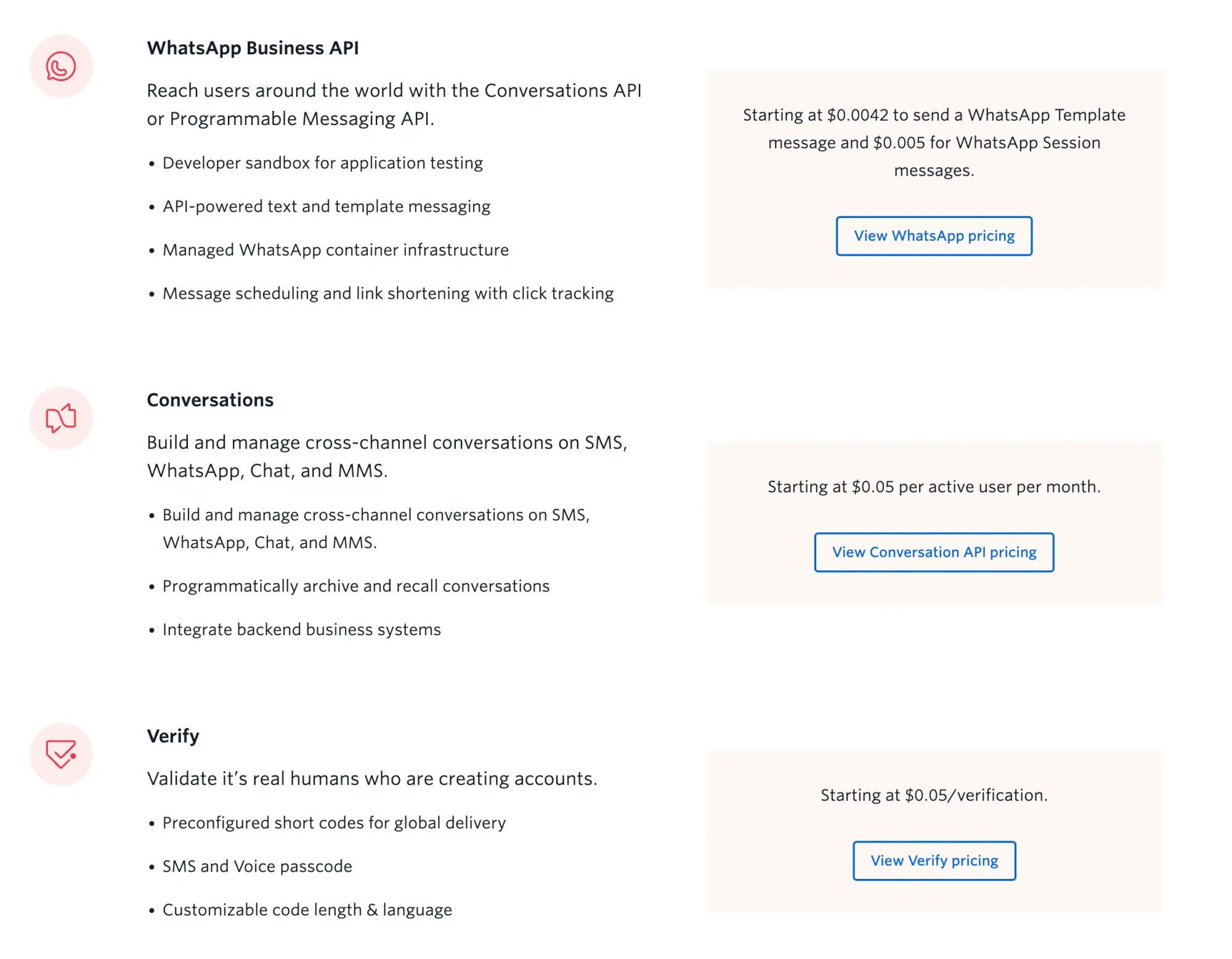
The reason they do this type of pricing is because others use Twilio to build things with them. The only truly new model might be success-based pricing: The idea that customers pay per successful thing the AI did. This, however, won’t apply to all of them.
Perhaps AI changes everything—just not pricing.